Discovering and exploring cases of educational source code plagiarism with Dolos — 2024
SoftwareX
Abstract
Source code plagiarism is a significant issue in educational practice, and educators need user-friendly tools to cope with such academic dishonesty. This article introduces the latest version of Dolos, a state-of-the-art ecosystem of tools for detecting and preventing plagiarism in educational source code. In this new version, the primary focus has been on enhancing the user experience. Educators can now run the entire plagiarism detection pipeline from a new web app in their browser, eliminating the need for any installation or configuration. Completely redesigned analytics dashboards provide an instant assessment of whether a collection of source files contains suspected cases of plagiarism and how widespread plagiarism is within the collection. The dashboards support hierarchically structured navigation to facilitate zooming in and out of suspect cases. Clusters are an essential new component of the dashboard design, reflecting the observation that plagiarism can occur among larger groups of students. To meet various user needs, the Dolos software stack for source code plagiarism detection now includes a self-hostable web app, a JSON application programming interface (API), a command line interface (CLI), a JavaScript library and a preconfigured Docker container. Clear documentation and a free-to-use instance of the web app can be found at https://dolos.ugent.be. The source code is also available on GitHub.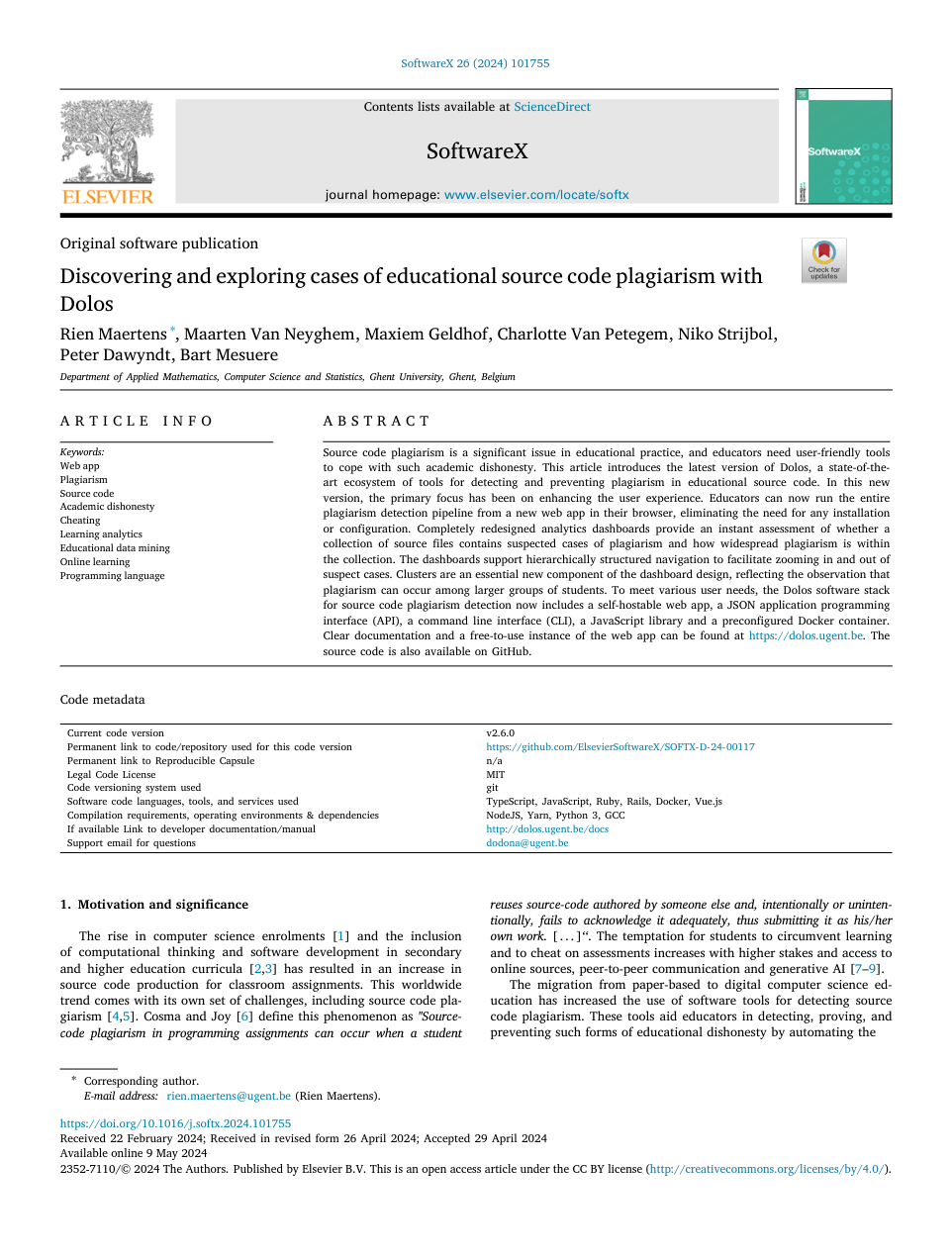